Disclosure: brianvanderwaal.com is supported by our users. Some of the links in this article may be affiliate links, which can compensate me at no cost to you if you decide to make a purchase.
Are you wondering how to get into AI? If you are exploring your career options or seeking a career change, this guide is for you.
Artificial intelligence, or AI, is one of the most exciting and rapidly growing fields in technology today. In 2025, as many as 97 million people will work in AI (Source: explodingtopics.com). With the potential to revolutionize industries and change how we live, it’s no wonder that many people are interested in entering this field.
In this blog article, I will cover everything you need to know about how to get into AI, from the basics of machine learning to the latest trends and developments. Whether you’re a student, a recent graduate, or a working professional looking to switch careers, this guide will provide you with the knowledge and resources you need to succeed in the exciting world of AI. So, let’s explore the world of AI and this career path together!
Who am I, and why should you trust my Career Information?
My name is Brian Vander Waal, and I am a Career Coach, Career writer, and career expert. I have invested more than 18 years in providing thousands of clients with career information, advice, and guidance. This article was written based on my career expertise, experience, and extensive research.
How to Get into AI: Skills for Success
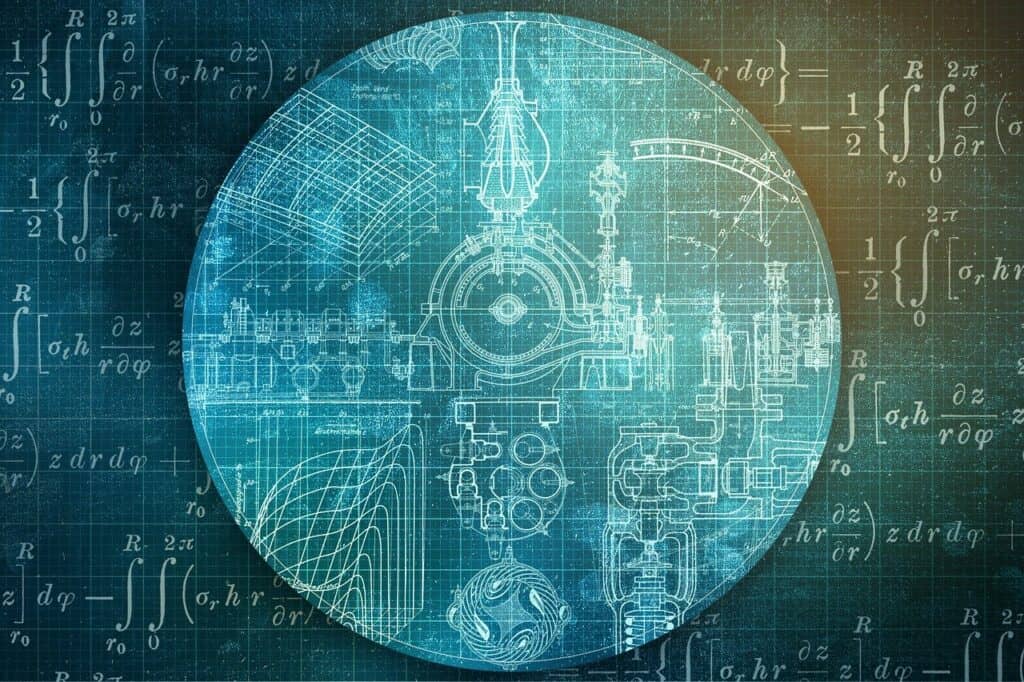
Breaking into the AI field requires a blend of technical skills, soft skills, domain expertise, and creativity. The key skills needed to succeed in AI are outlined below.
Programming Skills
Most AI applications require some level of programming expertise. Some of AI’s most commonly used programming languages include Python, R, and Java. Skills in version control (e.g., Git), unit testing, and designing scalable and maintainable code are invaluable.
Machine Learning
Machine Learning (ML) is a core subfield of AI. It is crucial to understand core machine learning algorithms and concepts like:
- Supervised Learning
- Unsupervised Learning
- Reinforcement Learning. (Source: Syndell).
Moreover, knowledge of Scikit-Learn, an open-source ML library for Python, is helpful.
Deep Learning
Deep Learning is a powerful branch of machine learning that utilizes artificial neural networks. Being familiar with Deep Learning frameworks like TensorFlow or PyTorch would be beneficial.
Natural Language Processing (NLP)
Understanding NLP techniques and libraries is essential for those interested in working with text data.
Mathematical Skills
Mathematics forms the backbone of many AI algorithms and techniques, including linear algebra, calculus, probability, and optimization methods. Here’s a more detailed look at why each of these areas of mathematics is crucial.
Linear Algebra
It is essential for understanding and working with data represented in multi-dimensional space. Concepts such as vectors, matrices, and tensor manipulations are foundational in neural networks and other machine learning algorithms.
Calculus
It is vital for understanding and implementing algorithms that involve optimization and change. Calculus is heavily used in backpropagation, a critical element in training neural networks.
Probability and Statistics
AI relies heavily on statistical analysis and modelling. A strong understanding of statistics is essential for developing accurate and reliable AI models. You need a firm grasp of probability and statistics to manage uncertainty, make predictions, and generalize from data. Bayesian methods, hypothesis testing, and statistical models are some examples of where this knowledge is applied.
Optimization Methods
These methods help find the best solution or make the best decisions within a defined set of possible solutions. Gradient descent and other optimization algorithms are at the core of many machine learning techniques.
Data Engineering Skills
Working with AI often involves large datasets, so data engineering is a crucial skill within the industry. Data engineering skills like data cleaning, manipulation, and preparation are essential for building robust AI models. More specifically, expertise in data preprocessing and transformation and proficiency in tools such as Pandas, NumPy, and SQL are vital.
Algorithm creation methodologies
AI professionals must be adept at creating, understanding, and modifying algorithms to solve specific problems. This involves knowledge of various algorithms, such as the ones used in machine learning, neural networks, and reinforcement learning.
Domain Expertise
Domain expertise is important for anyone working in AI, as AI applications are often tailored to specific industries and domains. For example, a data scientist working in healthcare will need to have a strong understanding of medical terminology and practices.
Cloud Computing
Many AI applications are deployed on cloud platforms. Understanding cloud platforms like Google Cloud Platform (GCP), Amazon Web Services (AWS), or Microsoft Azure would be valuable.
Strong Analytical Skills
AI professionals need to be able to analyze large sets of data, identify patterns, and derive meaningful insights to guide the development of AI models. Analytical skills are critically important for a career in AI.
Problem-solving skills
AI projects often involve complex, unstructured problems that require innovative and efficient solutions. Approaching problems methodically and creatively is essential for developing effective AI systems.
Creativity
While AI and machine learning are grounded in rigorous scientific principles, creative thinking can lead to groundbreaking applications and novel approaches to existing problems. Creative skills are crucial for developing new ideas, exploring unusual methodologies, and pushing the boundaries of what AI can achieve.
Summary
Building a career in AI involves acquiring these skills and applying them to real-world projects. It also consists in staying updated with the latest research and advancements and continuously learning new technologies and methodologies as the field evolves. Further, one must understand the nuances of data and ensure that AI solutions are based on accurate and relevant information.
Professionals who continue to develop these skills will be well-equipped to navigate the rapidly changing artificial intelligence industry. With a combination of these skills, you’ll be on your way to a bright future in AI. Remember, practice, curiosity, and continuous learning will fuel your success in this exciting field!
How to Get Into AI: Career Paths
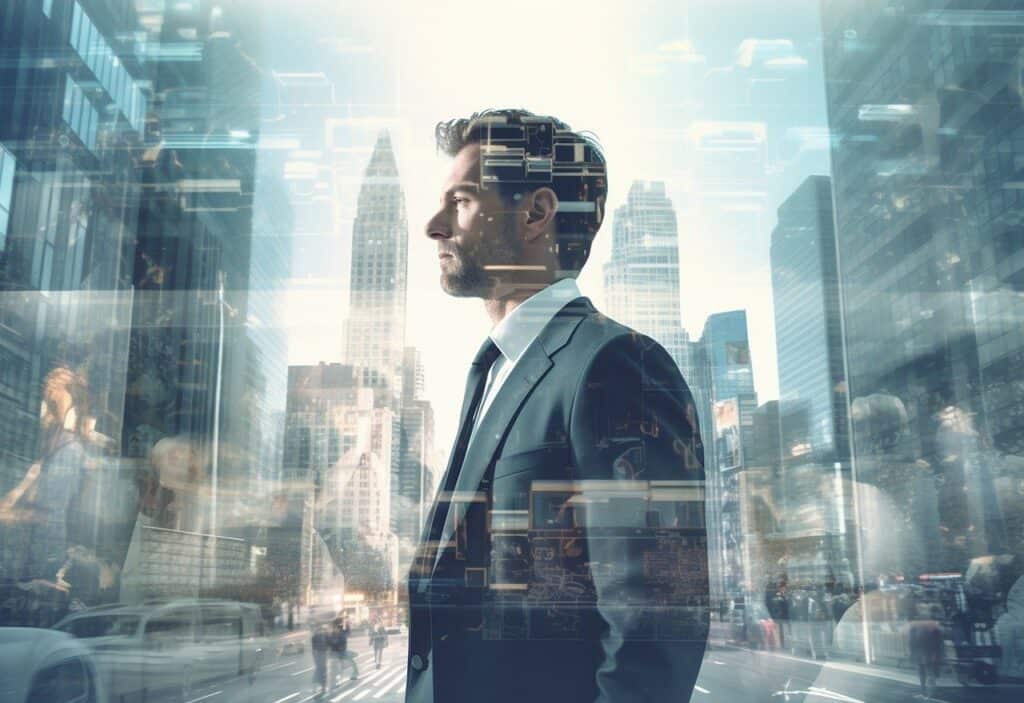
Are you curious about using your skills to participate in the AI revolution?
Learn about 11 different AI career paths, the skills you’ll need to succeed, and how to take that first step towards an incredible future.
1. AI Engineer
An Artificial Intelligence Engineer is responsible for crafting, programming, and training intricate networks of algorithms that mimic the functioning of the human brain. AI engineering demands diverse skills, including software development, programming, data science, deep learning, computer vision, and data engineering.
In addition to these technical skills, an AI engineer requires soft skills, including strong analytical skills, innovative problem-solving skills and a creative mindset to conquer complex challenges and meet organisational demands.
While AI engineers share some similarities with data engineers, their primary focus lies in:
- Leveraging data from various sources
- Constructing and refining machine learning model/models
- Deploying an AI application/applications using APIs or embedded code.
2. Machine Learning Engineer
Machine learning engineers primarily build, train, and deploy machine learning models. Their expertise lies in the algorithms and tools machines use to learn from data.
It’s important to note that Machine Learning Engineers are a vital subspecialty within AI Engineering. They focus extensively on developing and implementing machine learning algorithms.
3. Data Scientist
On the other hand, a Data Scientist must become proficient in a range of machine learning algorithms. Mastery of supervised learning models and unsupervised learning models / unsupervised clustering algorithms is essential for extracting valuable insights from vast datasets. With technical expertise and a solid understanding of statistics, data scientists can develop accurate AI models that drive meaningful results.
4. Robotics Engineer
Robotics Engineers design, build, test, and program robots to perform various tasks. They create robots with AI capabilities, enabling them to interact with their surroundings, learn, and make decisions autonomously. You’ll need a strong foundation in mechanical engineering, robotics, and programming languages like Python or C++ to thrive in this role. Additionally, knowledge of control systems, sensors, and computer vision is highly sought-after.
5. Natural Language Processing (NLP) Engineer
Have you ever interacted with a chatbot that understands your questions? That’s the magic of Natural Language Processing! NLP Engineers specialise in designing and developing systems that enable computers to both understand and also process human language. This field is crucial for AI applications like chatbots, voice assistants, and machine translation. You’ll need linguistics, machine learning, and deep learning expertise to excel as a Natural Language Processing Engineer. Additionally, expertise in programming languages like Python and familiarity with NLP frameworks like spaCy or TensorFlow are valuable assets.
6. System Engineer
Systems Engineers possess in-depth knowledge of how machines learn from their environment. They work with computer vision systems and natural language processing programs to solve intricate problems using autonomous agents. Their expertise enables the development of cutting-edge AI applications that can perceive, understand, and interact intelligently with the world.
Mastering these core skills equips individuals with the foundation to pursue a successful career in AI. By staying updated on the latest advancements and understanding what employers seek in this competitive field, aspiring AI professionals can position themselves as valuable assets to organisations at the forefront of technological innovation.
7. AI Developer
An Artificial Intelligence developer is responsible for incorporating AI functionality into software applications. This role focuses on integrating and implementing AI algorithms and logic into IT projects. To excel as an AI developer, you need cloud experience, programming expertise and knowledge of coding languages like Java, Python, and R.
In addition to these technical skills, employers often seek soft skills in AI developers, including problem-solving, critical and logical thinking, leadership abilities, strong decision-making skills, effective communication, collaboration, and emotional intelligence. These qualities are valued across industries and contribute to professional success in any position.
8. AI Researcher
For an Artificial Intelligence Researcher, grasping various computer science development techniques, including coding languages like Python or C++, is crucial. These fundamentals lay the groundwork for creating innovative AI algorithms and solving complex problems precisely. Additionally, familiarity with basic brain science provides valuable context, as it sheds light on the cognitive processes underpinning human and machine intelligence.
9. Product Manager
Product Managers are vital in creating successful Artificial Intelligence products that meet customer requirements and align with business objectives. Their extensive knowledge of market insights and technical expertise enables them to identify opportunities and design innovative solutions that leverage AI technologies effectively.
10. AI Security Specialist
AI Security Specialists identify and address vulnerabilities in AI systems. They work to prevent cyberattacks and ensure the security of data used to train AI models. To succeed as an AI Security Specialist, you’ll need cybersecurity expertise, knowledge of AI vulnerabilities, and an understanding of data security best practices. Soft skills like problem-solving, analytical thinking, and staying up-to-date on evolving threats are also crucial.
11. AI Ethicist
As AI becomes more sophisticated, ethical considerations become paramount. AI ethicists ensure that AI development and implementation align with ethical principles. This role involves identifying and mitigating potential biases in AI, ensuring fairness and transparency in AI applications, and promoting the responsible use of AI technology. You’ll need a strong understanding of AI principles, ethics, and philosophy to excel in this field. Additionally, knowledge of relevant laws and regulations surrounding AI is a plus.
The Future is Now: Are You Ready to Help Shape It with AI?
The AI revolution is creating a wealth of exciting career paths, each with the potential to make a real difference. Now is the time to take action. Embrace the future and discover how you can make a difference in the world of AI. Explore these career paths, enhance your skills, and join the revolution that is transforming industries and improving lives.
How to Get Into AI: Education and Training Requirements
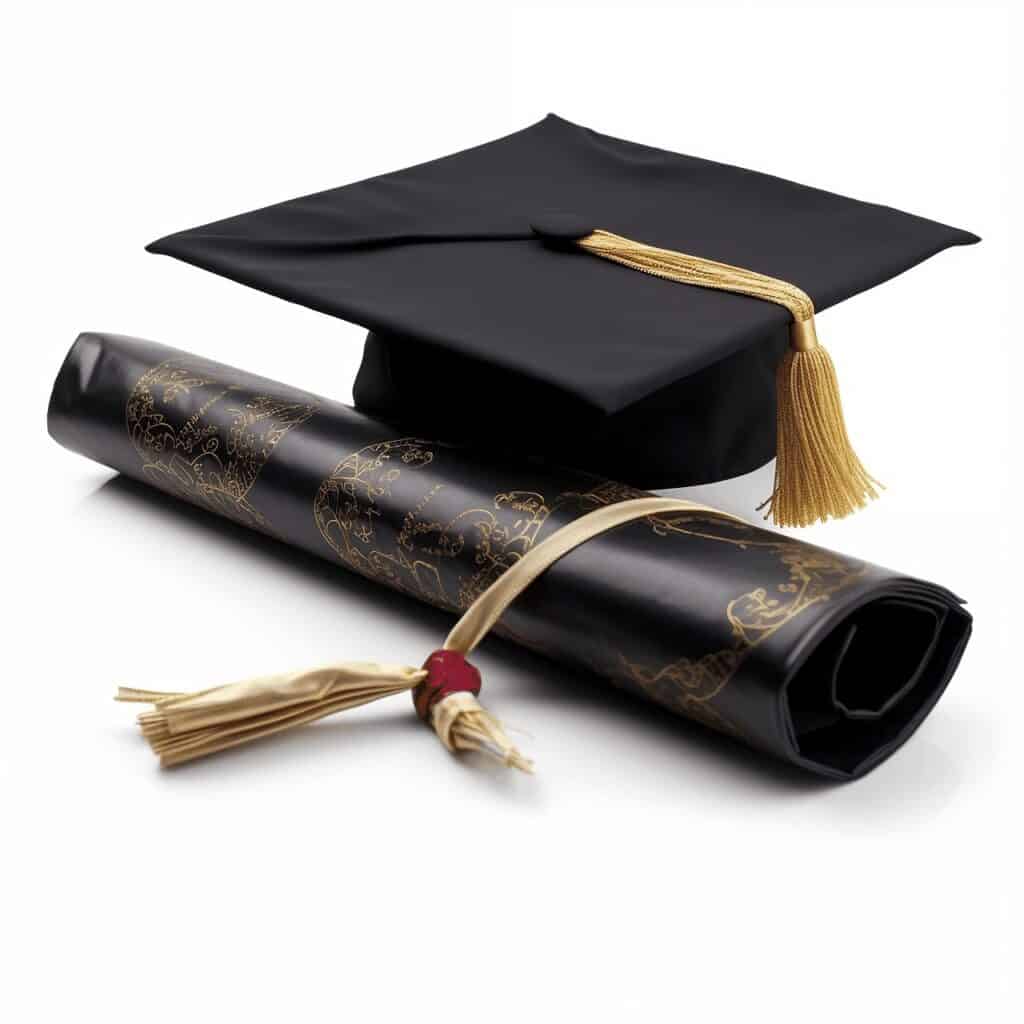
AI is an exciting and highly sought-after profession today. To become an AI engineer, start with a strong educational foundation. A solid foundation in mathematics, computer science, and engineering is vital for success in learning AI and within the field. Familiarity with machine learning algorithms and programming languages like Python gives you a competitive edge. Explore introductory Python courses and study existing AI tools. Expand your skills with online courses covering machine learning, data science, and more. These courses provide the knowledge and skills necessary for higher education and employment success.
High School
Build your AI career by laying the groundwork in high school with subjects like mathematics, physics, and computer science. These subjects will help you when you learn AI.
University
A degree or master’s in Computer Science, Data Science, or a related field is often the most direct path to a career in AI, as these degrees provide a strong foundation in programming, statistics, and other key skills. Many universities now offer specialized degrees in AI and Machine Learning (ML), which can provide even more focused training.
Apprenticeship
If you are looking for more intensive experience, consider an apprenticeship to tackle real-world AI projects using cutting-edge technology. Apply what you have learned through practical projects available on public cloud platforms for machine learning.
Certifications
Certifications in data science and AI technologies, such as machine learning or natural language processing, can also be useful for demonstrating expertise in a particular area of AI. Many certification programs are available online and can be completed in a matter of weeks or months.
Boot Camps and Other Training Programs
Boot Camps and other training programs can also be valuable ways to gain hands-on experience with AI technologies and learn from industry experts. Many Boot Camps focus specifically on AI and machine learning, providing a comprehensive and immersive learning experience.
How to Get Into AI: Upskilling Yourself in AI
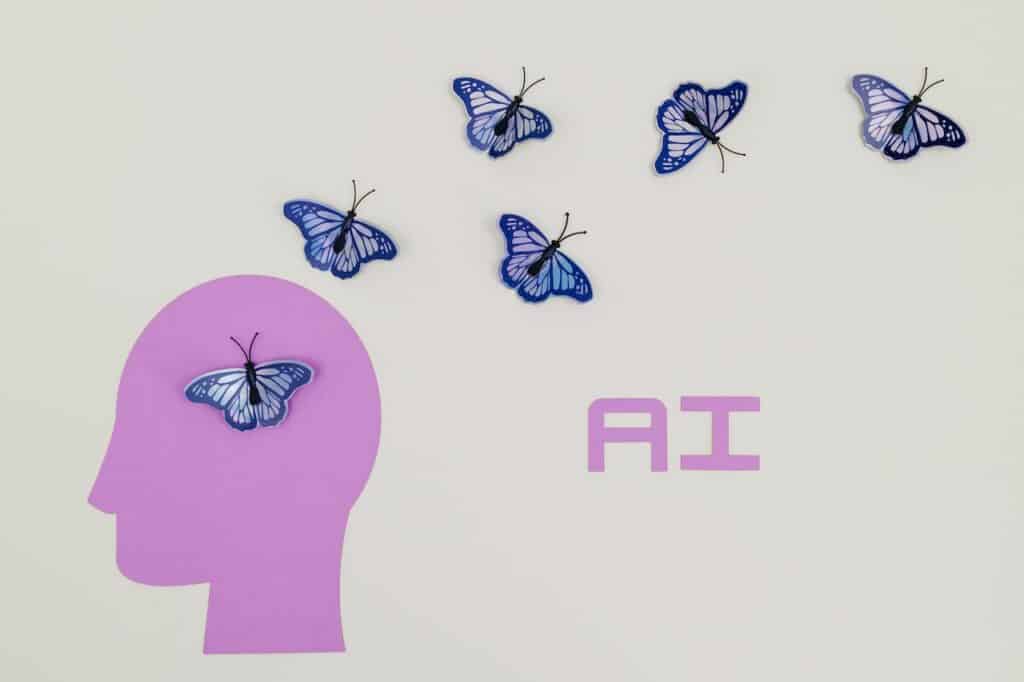
In today’s job market, staying ahead means upskilling in AI. Investing in continuous education is essential to stay competitive. Take courses to become a desirable candidate for higher positions. Continuous learning can even lead to management roles within organizations. It’s essential to grasp the latest advancements, current trends and technologies to ensure success in this career path.
I recommend that you enrol in an online course through platforms like Coursera, Edx, Udemy, Udacity and Pluralsight for detailed courses and real-world projects. Consider courses in Python, data science, deep learning, natural language processing (NLP), computer vision, robotics engineering and machine learning techniques, such as neural networks and clustering algorithms. Understanding these topics is invaluable for job applications, and these courses will equip you with the necessary skills and practical experience.
Don’t forget to stay updated. Attend AI seminars and conferences, and join online forums to understand employer expectations and network with peers. The demand for AI skills extends beyond tech companies, so be prepared to apply your knowledge in various industries.
Upskilling in AI gives you a competitive advantage. Explore the right tools and make well-informed choices for your professional journey. Embrace the exciting world of AI and position yourself for success!
AI Job Market Outlook
The AI job market is currently one of the hottest and fastest-growing in the world, with demand for AI talent far outstripping supply. Artificial Intelligence is on the rise and showing no signs of slowing down, presenting a wealth of chances for those with appropriate abilities. The US Bureau of Labor Statistics predicts that in the next 10 years, AI jobs will grow nearly five times faster than the average for all other occupations.
According to the World Economic Forum’s Future of Jobs Report, it is projected that 85 million jobs could be lost due to changes in labour division between humans and machines by 2025. However, the same period could see the creation of 97 million new AI jobs. Moreover, according to Business Wire, in Quarter 2 of 2024, there were 29,871 vacancies in artificial intelligence jobs, a 5.6% increase from Quarter 1 of 2024 and a 31.5% increase from the same period in 2023. Many experts believe this rapid growth will continue.
AI Career Salary Expectations
AI has transformed how we do business, making it a key component of numerous job roles. Professionals working in the field of AI can expect to earn competitive salaries and enjoy a high degree of job security. AI engineers are in high demand, with salaries ranging from $114,000 to $765,000 depending on location and experience. In the UK, graduates receive a starting salary of around £35,000, but this can increase to £170,000 if you work for a company like Google or Facebook (Prospects).
The median pay for Artificial Intelligence jobs in the USA is $157,196, or $75.57 hourly (businesswire.com). In the UK, the average salary for AI Engineers is £56,614 per year (Glassdoor).
Some of the most in-demand AI jobs include data scientist, machine learning engineer, and AI researcher. Salaries for these roles can range from $80,000 to $250,000 or more, depending on experience and location.
Applied AI: From AI Theory to Real-World Impact
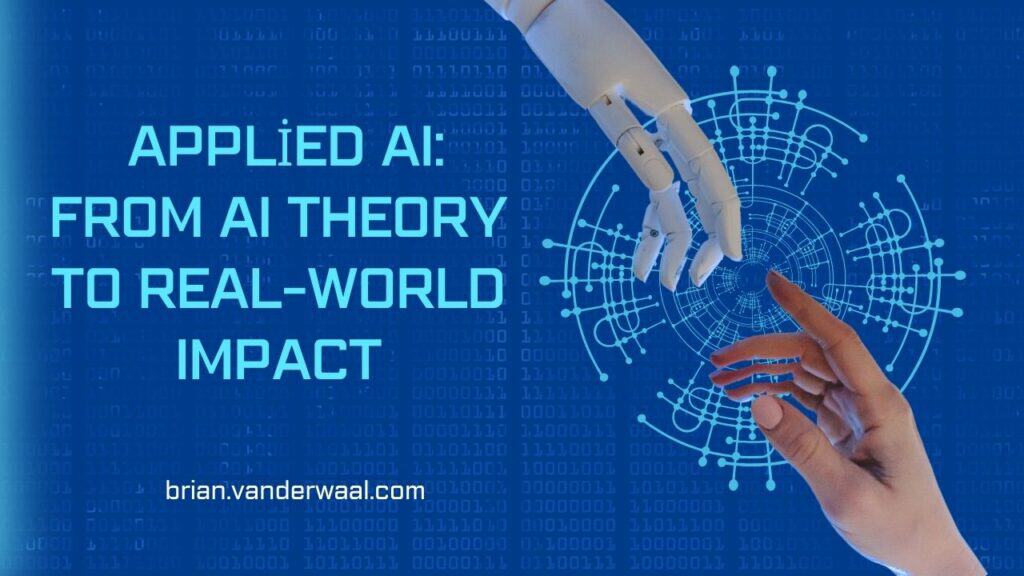
What is Applied AI?
Applied AI is all about practicality. It’s not about dreaming up fancy algorithms, but about using existing tools and technologies to solve real-world problems. It’s like turning a brilliant idea into something tangible and useful. This is the bridge between the crazy-smart AI researchers and the doers who make things happen.
Let’s say you’re a doctor trying to find a cure for a disease. Applied AI isn’t about creating a robot doctor (though that would be cool). It’s about using AI to analyze medical data, spot patterns, and suggest potential treatments.
That’s applied AI in action – solving a real-world problem with technology.
Essential Skills for Applied AI
As a Machine Learning (ML) Engineer, you need advanced knowledge of computer science, programming languages like R, TensorFlow frameworks, machine learning algorithms, and data structures to create innovative solutions.
Understanding of how machines learn are also crucial for success in applied AI. Further, experience with databases, software engineering, and data analytics is valuable.
However, being an Applied AI whiz is more than just cranking out code. Sure, knowing your way around Python and R is a must, but the blend of tech smarts and problem-solving creativity really sets you apart.
Imagine being a detective. You need to understand the crime scene (your data), gather clues (algorithms), and piece together a story (your model). That’s essentially what an Applied AI engineer does. You’re constantly curious, digging deeper into the data to uncover hidden patterns. And when things don’t go as planned (which happens a lot in AI), you’re the one who figures out why and fixes it.
So, while those fancy algorithms and programming languages are essential, remember the human side. Your ability to think critically, collaborate with others, and tell a compelling story with your results is just as crucial.
Building a Career in Applied AI
So, you want to build a career in Applied AI? Awesome! There are a bunch of ways to get started. To pursue a career in applied AI, you can take online courses, pursue a master’s degree in Computer Science or Information Technology focusing on Machine Learning or Deep Learning, or obtain certifications in specific disciplines like NLP or Computer Vision.
But here’s the thing: book smarts are just the beginning. The real benefits come when you start playing around with the tools. You have to get your hands dirty with real-world projects. That means understanding how to use cloud platforms like AWS, Azure, or GCP. They have many resources and pre-built models that can supercharge your AI projects. It’s like having a team of AI helpers ready to assist you.
Remember, Applied AI is all about solving problems. So, the more tools you have in your belt, the better equipped you’ll be to tackle any challenge that comes your way.
Tools and Technologies for Applied AI
Building cool AI stuff isn’t about reinventing the wheel. You’ve got a whole toolbox of awesome tools at your disposal. Languages like R are your trusty workhorses, crunching numbers and making sense of data. R is great for statistical analysis and data manipulation, providing a solid foundation for any data-heavy task. And let’s not forget about Python. Python is highly adaptable and can be utilized for a wide range of tasks, including data science and web development.
Then there are the cloud giants – AWS, Azure, and GCP (which I introduced above). These platforms are like having your own army of computers, ready to tackle the toughest AI challenges. Need to train a super complex model? No problem. They’ve got you covered.
And finally, we have the open-source heroes: TensorFlow, PyTorch, and Scikit-learn. These tools are like open cookbooks, sharing their secrets with anyone who’s curious. You can build on top of what others have created, saving you time and effort. It’s like standing on the shoulders of giants but with better code.
The Importance of Practical Experience
Embarking on applied AI projects helps gain practical experience and develop the necessary skills. Let’s face it, AI is cool, but it’s also really complex. The best way to grasp it is by diving headfirst into real-world projects. It’s like learning to swim – you can read all the books you want, but nothing beats getting in the water.
Building your own AI projects is the best way to learn. You’ll run into challenges, make mistakes, and learn from them. And that’s precisely what you need to grow as an AI pro. Plus, having a portfolio of projects to show off is a huge plus when job hunting.
Oh, and one more thing: don’t just focus on the tech side. To truly grasp AI, you must understand how the human brain works. This is where Brain Science comes in. Understanding how humans make decisions, learn, and adapt can help you build AI systems that are more human-like. It’s like reverse engineering the human mind, which is both super cool and totally mind-boggling. By exploring psychology and cognitive science, you can create AI systems that are more intuitive and user-friendly. I discuss this further in the next section below.
Why is Understanding Brain Science Important in AI Careers?
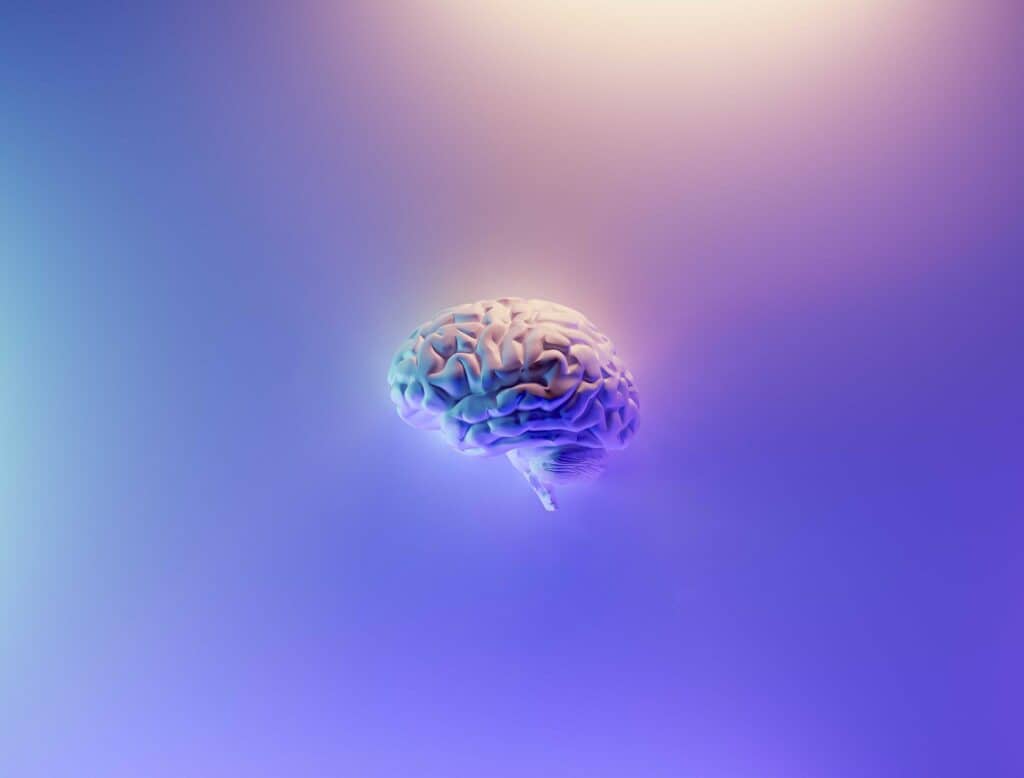
So, why is understanding Brain Science important in AI Careers? I mentioned above that if you want to truly grasp AI, you must understand how the human brain works.
Understanding the inner workings of the brain is key for those venturing into the world of Artificial Intelligence. Think about it—our brains are decision-making powerhouses, and AI is all about trying to replicate that genius. To create smart AI solutions that truly mimic human thinking, studying and exploring brain science is crucial.
By peeling back the layers of how the brain works, we can build AI algorithms that factor in real-world variables like traffic patterns and environmental changes. The aim is to craft AI systems that think and decide as we do. Understanding human thought processes is the key to success in this field.
Picture this: you’re designing an AI system for self-driving cars. It’s not just about programming it to follow traffic rules. You want it to make decisions like a human driver would, considering things like road conditions, weather, and even the behaviour of other drivers. That’s where brain science comes in handy.
Now, I know what you’re thinking. “Brain science? Isn’t that super complicated?” Well, yeah, it can be. But don’t worry! There are some awesome courses out there that break it down for us non-neuroscientists.
I’ve been checking out some Brain Science classes on Coursera and Udemy. You learn about cognitive development, machine learning algorithms, and how our brains process information. With this knowledge, you’ll be ready to build robust applications backed by machine learning and succeed in AI.
And here’s the kicker – this knowledge isn’t just cool to have. It’s becoming essential in the AI field. As AI keeps spreading its tendrils into different industries, understanding the brain is vital.
Think about it. When you’re designing an AI system, wouldn’t it be awesome to know how humans actually process information? It’s like having a cheat sheet for creating more intuitive and effective AI.
Plus, let’s be real – the AI field is getting pretty competitive. Having this brain science knowledge under your belt? It’s like having a golden ticket. It shows potential employers that you’re not just following the crowd, but really thinking about the foundations of AI.
So, here’s my advice: try a brain science course. Trust me, it’ll add so much context to your AI work. You’ll start seeing connections everywhere – in computer vision, natural language processing, you name it.
Bottom line? If you want to stay ahead in the AI world, understanding brain science is the way to go. Completing a brain science course doesn’t just broaden your horizons—it gives you a competitive edge when applying for AI jobs or internships. It’s not just about keeping up with trends – it’s about setting them.
However, having a knowledge of Brain Science and Applied AI is useless without the right tools. We will explore that next.
AI Tools and Resources to Try if You Want to Get Into AI
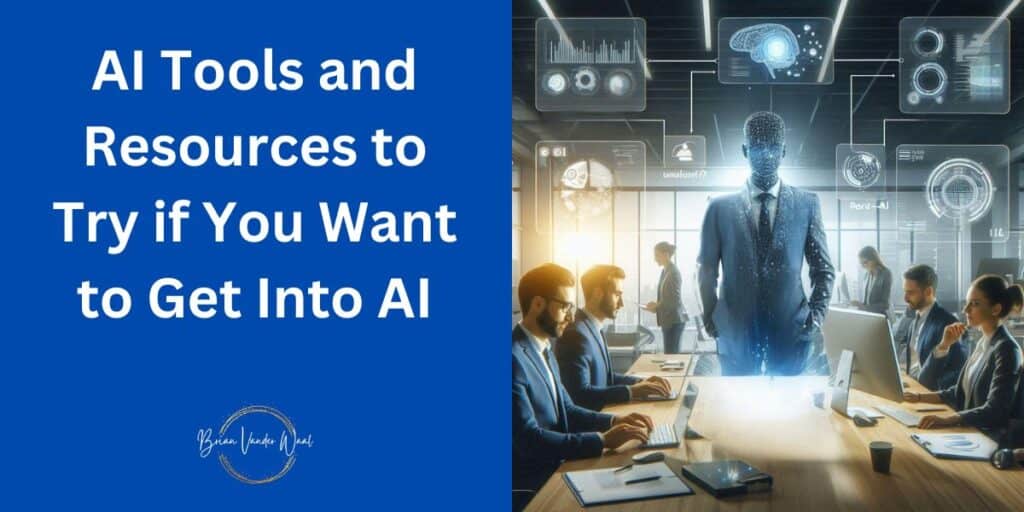
The allure of building intelligent AI systems is undeniable, but breaking into the AI field can feel like trying to navigate a dense fog without a compass. With a plethora of tools, libraries, and platforms available, finding the right starting point is crucial. Luckily, there are some great choices that’ll set you on the right path. I will guide you through the best AI tools and resources to try if you want to get into AI.
I will cut through the noise and provide a clear path forward. Discover the essential tools, platforms, and learning resources that will kickstart your AI journey. Whether you’re a seasoned developer or just starting out, there’s something here for everyone.
1. Cloud Service Providers
When exploring the best AI tool or tools, remember it is not just about programming languages. Begin your AI adventure by exploring public cloud providers like Google Cloud, Amazon Web Services (AWS) and Microsoft Azure. These platforms offer a wide range of services for researchers. They have all sorts of documentation and tutorials that cover everything from basic machine learning to advanced topics like deep learning. And the best part? You can try out code using Jupyter Notebooks without having to set up any complicated software on your own computer.
Cloud services also provide a way for developers to build, deploy, and scale AI applications in the cloud. These services often include pre-built AI models and algorithms and tools for data storage, processing, and analysis. They let you leverage the latest advancements in AI, such as machine learning and deep learning, to test out your ideas and gain a comprehensive understanding before embarking on your own projects.
2. Research Papers and Reports
Ever wonder how tech giants like Google and Facebook do their AI magic? Well, they’re not as secretive as you might think. They often share research papers about their work on AI that give us mere mortals a peek behind the curtain.
Reading through these reports can give you a glimpse into how these companies tackle problems using machine learning and other strategies. You’ll get a feel for what it takes to build successful AI products and stay up to date with the latest industry trends.
3. Open-source Libraries
To sharpen your skills even further, check out web-based tools that can help you refine your expertise. Open-source libraries, such as TensorFlow and PyTorch, provide a wealth of pre-built algorithms and models that can be customized and used in a wide range of applications. They are like the Lego blocks of AI. These libraries are often free and open to the public, making them a valuable resource for researchers and developers.
Techniques like TensorFlow and PyTorch are great for data science, natural language processing (NLP), and reinforcement learning (RL). These resources are accessible to anyone, allowing you to become an AI expert in no time.
4. Application Programming Interfaces (APIs)
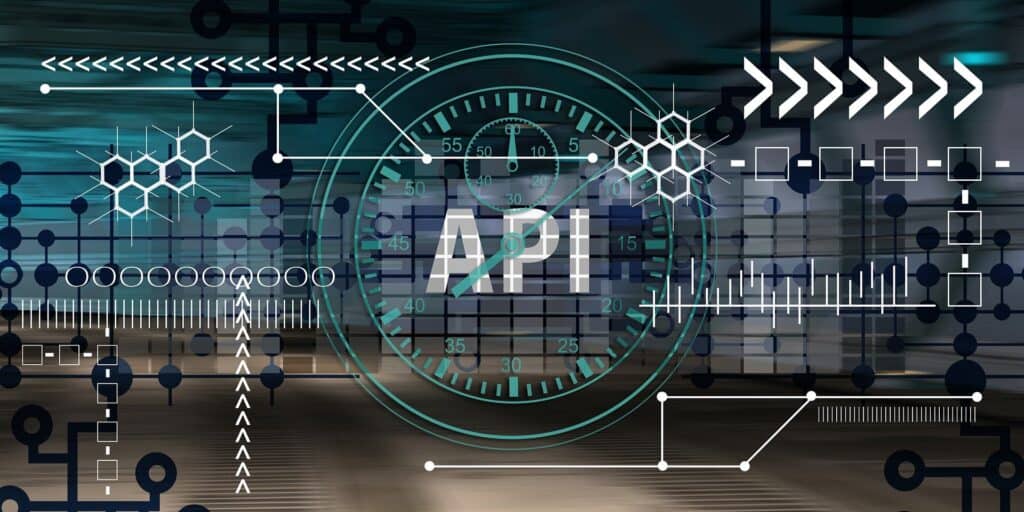
APIs, or application programming interfaces, allow developers to access pre-built AI services and functionality. APIs are often provided by AI vendors and can be used to add AI capabilities to existing applications or build new applications from scratch.
Ethics and Challenges in AI
As AI becomes more advanced and widespread, it is crucial to consider this technology’s ethical implications and challenges. Some key ethical concerns in AI include bias, privacy, and regulation.
Bias
Bias is a major concern in AI, as algorithms can inadvertently perpetuate and amplify existing biases and inequalities. For example, facial recognition algorithms have been shown to be less accurate when identifying people of color, which could have serious implications for the use of applications.
Privacy
Privacy is also a concern in AI, as many AI applications rely on large amounts of personal data. It is important to ensure that this data is collected and used responsibly and that individuals have control over how their data is used.
Regulation
Regulation is another critical issue in AI, as the technology is still largely unregulated in many parts of the world. It is important to develop frameworks and regulations to ensure the safe and responsible development and use of AI.
Future of AI and its Impact on Society
AI’s future is exciting and uncertain, with the potential for transformative change and significant challenges. Some of the key trends and developments in AI include the rise of deep learning and neural networks, the growth of AI-powered automation, and the increasing focus on ethical considerations and responsible development.
AI can potentially transform every aspect of our lives, from healthcare to transportation to entertainment. However, it is important to ensure that these changes are made responsibly and with consideration for the potential risks and challenges.
Conclusion
Now is the perfect time to dive into the exciting world of AI! Whether you’re a student, a recent graduate, or a working professional looking to switch careers, there are many paths to success in AI. From developing technical skills to gaining domain expertise, there are many ways to break into the field and make a meaningful impact.
Equip yourself with the right skills and knowledge, stay updated on the latest trends, explore job opportunities, connect with industry experts, and invest in your education. With the right qualifications and certifications, you can build a thriving career in Artificial Intelligence. Acquiring the necessary skills, understanding current AI trends, and staying informed about the latest advancements will make you a strong contender for any AI job.
Entering the world of AI may seem challenging, but it is absolutely attainable with the right expertise and capabilities. Numerous resources are available to help you research AI, courses that provide insights into brain science and AI, and many career opportunities waiting for you. To maximize your chances of success, dedicate time and effort to develop the necessary skills.
Frequently Asked Questions (FAQs) – How to Get Into AI
FAQ on the History of Artificial Intelligence
What is the History of AI?
Artificial intelligence has a long and fascinating history, dating back to the 1950s. Early AI projects aimed to create machines capable of human-like thinking and reasoning, but progress was slow. It wasn’t until the 1980s and 1990s, with advancements in computer hardware and software, that AI experienced a resurgence. Researchers began focusing on specialized forms of AI, such as machine learning and natural language processing, in their AI research, solving specific problems and tasks.
Today, AI is more advanced than ever before. Breakthroughs in deep learning and neural network have propelled rapid progress in AI development. From virtual assistants like Siri and Alexa to self-driving cars and facial recognition technology, AI is reshaping our relationship with technology and each other.
FAQ on the Main Types of AI
What are the Main Types of Artificial Intelligence?
There are seven main types of artificial intelligence, some existing now and others we believe could exist in the future.
1. Artificial Narrow Intelligence (ANI):
Artificial Narrow Intelligence (ANI) are specialized AI tools for specific tasks. They excel in one area but can’t learn beyond their programmed capabilities. Examples include virtual assistants like Siri and image recognition software.
2. Artificial General Intelligence (AGI):
Artificial General Intelligence (AGI) aims to create AI that can learn, think, and perform tasks like humans. It strives to be versatile and assist humans in various aspects of life using technologies such as supercomputers and generative AI models.
3. Artificial Superintelligence (ASI)
Artificial Superintelligence (ASI) is still in the realm of science fiction and represents AI surpassing human intelligence. It could outpace human abilities, but it’s currently speculative.
4. Reactive Machines
Reactive Machines are fundamental AI systems that react to immediate requests but lack memory or the ability to learn from past experiences. Examples include spam filters and recommendation algorithms.
5. Limited Memory AI
Limited Memory AI can store knowledge and improve over time. It learns from past experiences and represents the majority of AI applications today, from chatbots to self-driving cars.
6. Theory of Mind
Theory of mind refers to AI’s ability to perceive emotions and understand subtle environmental changes. While it is yet to be fully realized, it has the potential to revolutionize AI by allowing machines to interpret human emotions and predict actions.
7. Self-Aware AI
At the point of singularity, AI machines would possess self-awareness and the ability to sense others’ emotions. This stage remains hypothetical, with ongoing research and debate regarding its ethical implications.
FAQ on the Key Technologies of AI
What are the Key Technologies of AI?
Machine learning, natural language processing, computer vision, generative AI, and adaptive AI are key AI technologies that drive AI advancements.
1. Machine Learning
Machine learning enables machines to learn from data without explicit programming. ML algorithms analyze large datasets, identify patterns, and make predictions. They are used in diverse applications, from fraud detection to self-driving cars.
2. Natural Language Processing
Natural language processing helps machines understand and interpret human language. Analyzing text and speech identifies meaning, sentiment, and intent. Virtual assistants and chatbots rely on natural language processing.
3. Computer Vision
Computer vision enables machines to interpret visual data, identifying objects and people in images and videos. It is used in various applications, from security systems to self-driving cars.
4. Generative AI
Generative AI, also known as deep learning or neural networks, has become a prominent buzzword in the tech industry. It utilizes large datasets and complex algorithms to generate original content and has diverse applications, such as image recognition, speech processing, and art creation.
One of the significant impacts of Generative AI is its automation of complex tasks that were previously deemed beyond machines’ capabilities. Industries such as finance, healthcare, and legal can leverage Generative AI to analyze extensive data and make predictions, saving time and resources and reducing human error.
A prime example of Generative AI is ChatGPT, a language model developed by OpenAI. ChatGPT engages in human-like conversations, providing responses to various questions. Other Examples of Generative AI include Gemini, Copilot, DALL-E, Midjourney, and DeepMind.
5. Adaptive AI
Generative AI is not the final frontier. The next evolution is Adaptive AI, which combines advanced machine learning with real-time learning and responsiveness. Adaptive AI models constantly adapt and learn based on new data and feedback, making them highly versatile in dynamic work environments.
Adaptive AI’s ability to adapt to changing circumstances is advantageous in enhancing customer experiences and improving employee productivity. Tools like SubStrata exemplify adaptive AI capabilities by analyzing cues and emotions, facilitating faster decision-making.
With its responsiveness and flexibility, adaptive AI is poised to transform the digital workforce and empower companies to excel in the ever-changing marketplace.